Building a Future-Ready Customer Data Infrastructure: Types, Use Cases, and More
Today, brands can move beyond the traditional one-to-many personalization approach and deliver unique experiences tailored to each individual customer. While this is the goal of many organizations, you’re probably wondering—how do you get there?
81% of brands claim they have a deep understanding of their customers. But in reality, less than half (46%) of global consumers agree, according to Twilio’s State of Customer Engagement Report. This clearly indicates a gap in what brands perceive personalization to be in comparison to consumer expectations.
Imagine a customer walks into a physical store and purchases a pair of shoes. Later, they realize the shoes are uncomfortable and decide to initiate a return online. The customer logs into their account on the retailer's website, where the system recognizes their recent purchase. An intelligent virtual assistant pops up, already aware of the order details and ready to assist with the return process.
The virtual assistant guides the customer through the return steps, offers suggestions for alternative shoe styles based on the customer's preferences and purchase history, and arranges for the return shipping label to be sent via email. The customer selects a new pair of shoes recommended by the assistant, which are promptly shipped to their address.
After the new shoes are delivered, the customer receives a follow-up message through their preferred communication channel—whether it be email, SMS, or a mobile app notification—asking for feedback on the new purchase. Additionally, the message includes personalized product recommendations, leveraging the customer's entire shopping history to suggest items that align with their style and needs. This seamless integration of online and offline interactions creates a cohesive and highly personalized shopping experience.
Customer data has become an indispensable asset for organizations of all types. Effective data management strategies are paramount for businesses aiming to understand their customers deeply, enhance their experiences, and drive sustainable growth. This article delves into the various types of customer data, their practical use cases, and provides a comprehensive overview of customer data maturity to help you identify where you’re getting the most from your data and strategies.
Understanding Your Customer Data
What is Customer Data?
Customer data encompasses any information that businesses collect about their customers. This data is crucial for understanding customer behaviour, preferences, and needs, enabling companies to tailor their offerings and communications effectively. Effective use of customer data allows businesses to create personalized marketing strategies, improve customer engagement, and ultimately drive better business outcomes.
Types of Customer Data:
Zero-party Data: Coming Directly from the Customer
Zero-party data refers to information that customers voluntarily provide, such as preferences, opinions, and personal details shared through direct interactions. Businesses can collect this data through sign-up forms, surveys, preference centers, and quizzes. For example, a retailer might use a survey to ask customers about their favourite products and shopping preferences. This data is valuable for tailoring product recommendations and marketing messages based on explicit customer preferences.
First-party Data: Aggregated Behavioural and Transactional Data
First-party data is collected directly from customers’ interactions with the company’s own channels, such as websites, mobile apps, and in-store visits. This data includes web analytics, purchase history, loyalty and rewards status, and CRM data, which help build detailed customer profiles. For instance, a business might track browsing behaviour on its website to understand which products are most popular among different customer segments. This information can enhance marketing campaigns by targeting segments based on past behaviours and interactions, improving campaign return on ad spend, conversions, and more.
Second-party Data: Collected Through Partner Collaboration
Second-party data involves data exchanged with trusted partners, usually gathered by another organization with a similar audience and reliable data collection methods. Businesses can partner with complementary companies to share relevant data sets. For example, a fitness brand might partner with a health food company to exchange customer data that could help both brands target health-conscious consumers. This strategy allows companies to expand their reach and target new customer segments by leveraging data from similar but non-competing businesses. Check your relevant compliance requirements before diving into a second-party data initiative to ensure that consumers’ privacy choices, including explicit user consent, are respected.
Third-party Data: Widening Your Data Net
Third-party data is purchased from external sources that gather information from various platforms and sell it to other companies. This data can include demographic, geographic, and psychographic information. While third-party data can enrich customer profiles, it should be used with caution due to reliability and compliance issues, particularly concerning regulations like Canada’s Anti-Spam Legislation (CASL). For example, a company might purchase third-party data to refine audience targeting and improve ad placement strategies. However, the reliability of third-party data can vary, and it often lacks the precision and quality of zero-party and first-party data.
Customer Data Maturity Overview
With an understanding of your customer data types, you can begin evaluating where your organization is at with your overall customer data strategy. Creating outstanding customer experiences hinges on your customer data infrastructure. Organizations that efficiently leverage customer data are able to offer exceptional experiences, make informed business decisions, and achieve their growth objectives more effectively.
To gauge your data maturity and identify areas for improvement, focus on these key competencies: data collection, integration, activation, and adaptation.
What is Customer Data Maturity?
Customer data maturity refers to the extent to which an organization utilizes its customer data across all operations. The ultimate goal is to integrate customer data into decision-making processes to drive strategic growth and enhance customer experiences. A customer data maturity model helps businesses measure the sophistication of their data management operations, identify goals, challenges, and actions to take, as well as prioritize use cases for a customer data platform (CDP).
Benefits of a Customer Data Maturity Model:
Using a maturity model to assess customer data capabilities offers several benefits. It helps businesses set realistic goals for data management improvements, identify potential challenges, and outline actionable steps to enhance their data strategies. This strategic approach encourages organizations to adopt advanced data technologies, such as AI and machine learning, in a phased manner, ensuring they build a strong foundation before moving to more complex applications.
Stages of Customer Data Maturity
1. Initial Stage: Basic Data Collection and Storage
At the initial stage of customer data maturity, businesses primarily focus on the fundamental task of collecting and storing data from basic customer interactions. This might include data gathered from sales transactions, sign-up forms, and customer service interactions. For example, a retail store might collect customer names, contact information, and purchase histories through its point-of-sale (POS) system. This foundational data collection is crucial for building a database that can later be expanded and utilized for more advanced purposes, specifically for zero and first-party data collection.
2. Growing Stage: Integrating and Standardizing Data
As businesses progress, they enter the growing stage, where the emphasis shifts to integrating and standardizing data from various sources. This might include data from Customer Relationship Management (CRM) systems like Salesforce or HubSpot, marketing platforms like Klaviyo, and loyalty programs. By unifying these disparate data sources, businesses can create a comprehensive view of each customer.
For instance, an omnichannel retailer might integrate data from its POS system, which tracks customer interactions and purchase history, with data from its marketing platform, which monitors email campaigns and website engagement. Additionally, loyalty program data, capturing points earned and redeemed, can be combined to further enrich customer profiles. These integrations are essential for eliminating data silos and enhancing data accuracy. It ensures that all departments have access to the same up-to-date information, leading to more consistent and personalized customer experiences.
3. Maturing Stage: Analyzing Data for Insights and Customer Segmentation
In the maturing stage, organizations begin to analyze the collected data to gain actionable insights, identify trends, and segment their customers more effectively. By leveraging analytical tools, businesses can understand customer behaviour, preferences, and needs in greater detail.
Retailers can use this data to identify peak purchasing times, product and category insights for cross-selling, and preferred shopping channels (online vs. in-store). For example, a retail company might analyze purchase history and browsing behaviour to create targeted email campaigns tailored to different customer segments.
This stage is critical for transforming raw data into valuable insights that can drive strategic decision-making and long-term sales performance impact. By understanding customer preferences and behaviours at a granular level, the retailer can not only enhance the effectiveness of marketing campaigns but also improve product offerings, optimize inventory management, and ultimately foster stronger customer loyalty and increased sales.
4. Advanced Stage: Real-time Data Activation and Personalized Experiences
At the advanced stage of data maturity, businesses leverage real-time data to provide highly personalized customer experiences and proactive services. This stage involves using advanced technologies such as artificial intelligence (AI) and machine learning (ML) to deliver dynamic and personalized interactions. For instance, a public service portal might offer personalized recommendations and real-time updates based on user interactions and preferences. This capability enhances customer satisfaction and loyalty by ensuring that customers receive relevant and timely information and services.
Conclusion
Embracing a structured approach to customer data maturity ensures that businesses are well-equipped to meet the evolving needs of their customers and stay competitive in a data-driven world. By investing in the right technology, implementing effective data collection practices, and activating customer data to create personalized experiences, organizations can not only enhance customer satisfaction but also drive substantial business growth. In the end, a mature customer data strategy is not just a technical necessity but a critical driver of long-term success and innovation.
Stay informed, sign up for our newsletter.
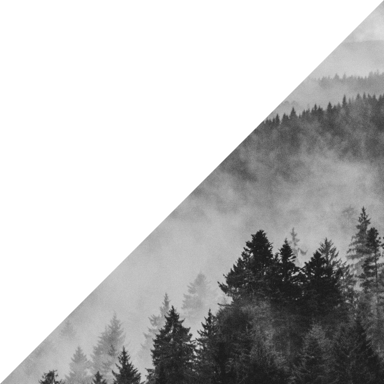